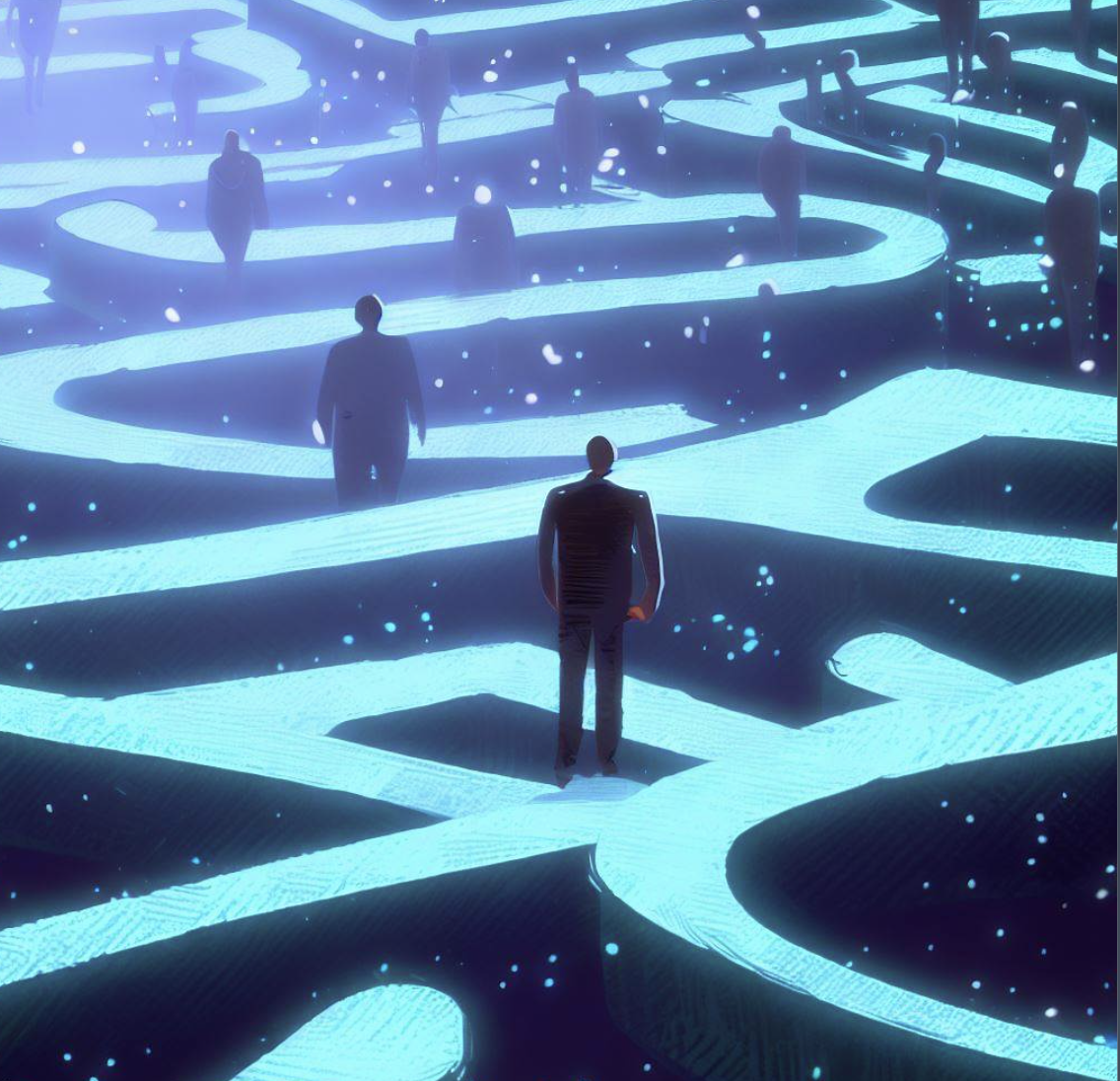
The diffusion of discovery
Contents
How do humans make discoveries? How do they venture into the unknown and push the frontiers of knowledge? In this article, we offer a new perspective on human discoveries, based on a simple but powerful analogy: humans are like particles diffusing out of a labyrinth. Imagine that each human is an explorer who tries to find an exit from a maze-like environment, where every turn is a gamble and every step is a risk. Some explorers are more adventurous than others, some follow the crowd, some learn from their mistakes, and some get lucky. As they explore, they gradually reveal the structure and size of the labyrinth, and reduce the fog of ignorance. This analogy allows us to model human discoveries as a stochastic process, and to quantify how fast and how far humans can diffuse in different scenarios. We can also use this model to derive some insights into the principles of scientific inquiry, such as the role of curiosity, collaboration, and diversity in fostering innovation and creativity. Of course, we do not claim that this model is perfectly accurate. It is primarily a toy model, a mental experiment, a way of thinking. But we hope that it can spark some interesting discussions and inspire similar models in the future.
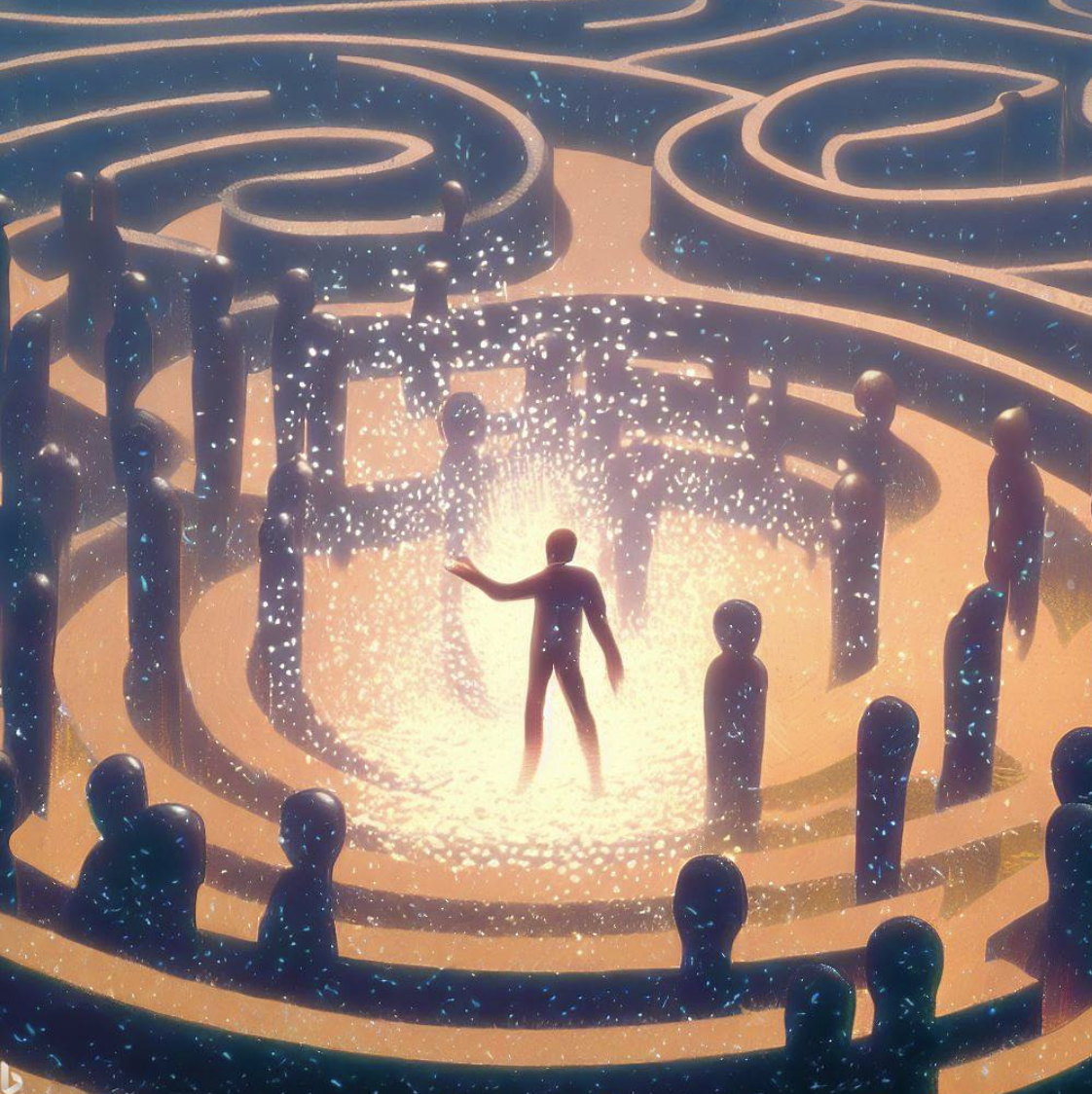
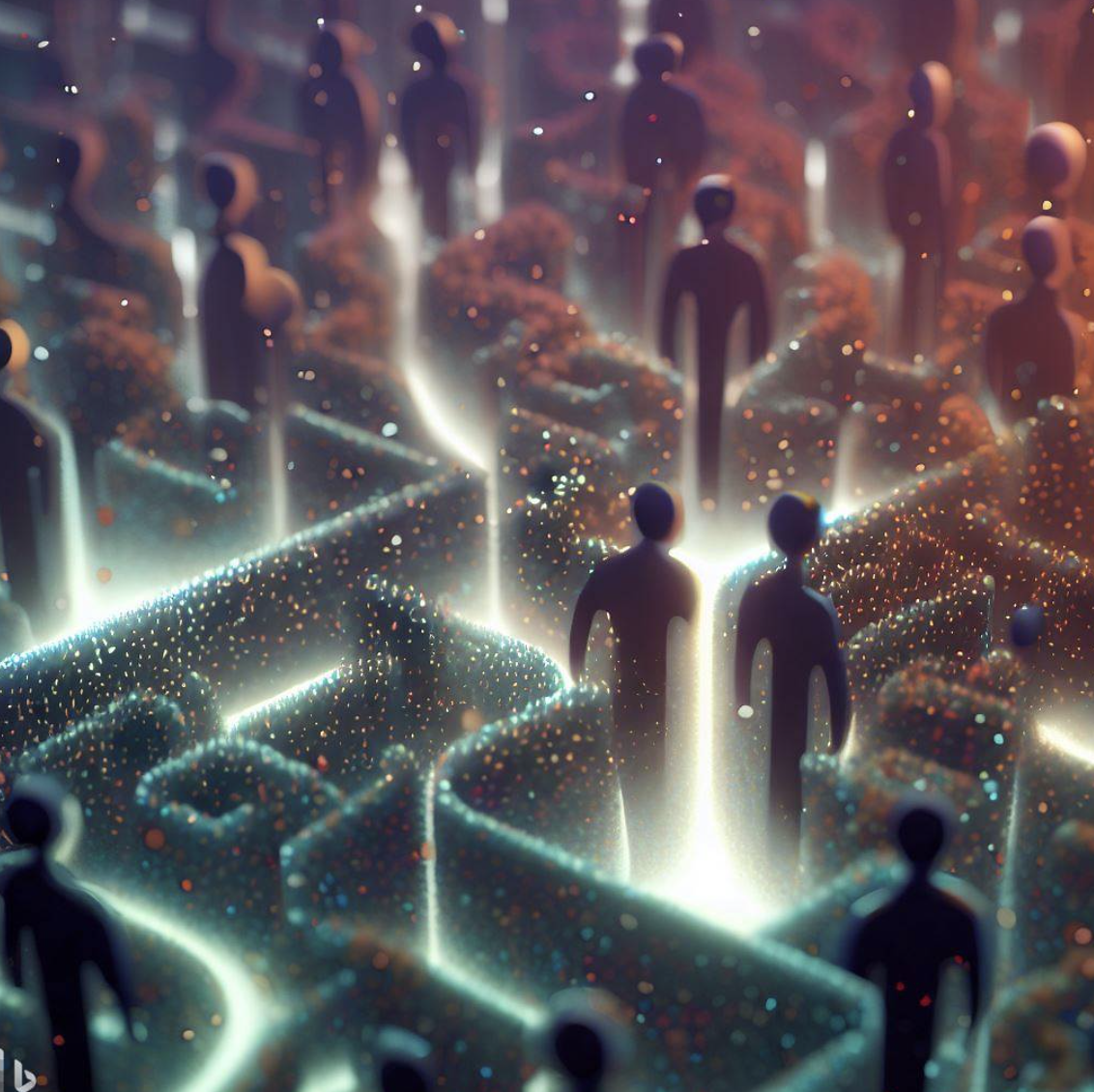
The diffusion process
If you want to start from scratch, first, click the restart button. You can start by experimenting with the various controls.
Escapism: 0.0005
Researchers: 100
Obstacles: 20
Most of the parameters are fairly self-explanatory. Escapism is the parameter that deserves some explanation. It characterizes the tendency to go “outwards”. These can be thought of as the researchers that take the “straight logical path” to the solution. It often works, but not always. The path to greatness is not always just a straight line. How about the opposite of an escapist? Someone who has $0$ escapism can be though of as someone who really does not leverage any structure into their research. They just hope to randomly come across the solution. Although it technically can happen, any random researcher is less likely to make it this way. In the above simulation, the parameter of Escapism still creates a range of researchers, with a maximum escapism based on the selection (the actual escapism corresponds to the shade of the particle).
Interpretations
-
This Labyrinth can represent either one domain of research, or research as a whole. It matches simple phenomena one would qualitatively expect: more researchers result in a higher rate of discoveries.
-
If someone makes a discovery, there’s often someone quite close to making the same discovery. This is often observed in scientific literature, where e.g. similar results are produced at the same time by multiple scientists.
-
Someone might stray far away from the average, making a breakthrough discovery after a long time. The longer it takes for a discovery to happen, the more likely this someone will be far ahead from the others (intepreted as more novelty).
-
After a big obstacle is overcome (the blue/grey arcs), there’s a lot of follow-up discovery that comes easily (but not always, depending on the next obstacle).
-
Obstacles researchers face have varying difficulty. Smaller opening of the arc means bigger the difficulty to overcome.
-
You might be at the frontier, but if you are working on the wrong problems or using the wrong approachs you will get stuck (far from the opening of the obstacle or heading the wrong way).
Implications
-
This model implies that someone making a discovery does not mean that without them the discovery would have never been made. This someone’s contribution lies in the reduction of the interdiscovery time. These implications are compatible with insights from books such as Outliers, Sapiens, and Fooled By Randomness. To state it in another way: a discovery is an accident waiting to happen.
-
If we are merely “particles” performing a random walk, then what control do we have? How can we have any merit in our discoveries and any credit for them? Controlling the properties of this biased random walk to perform a more intelligent “search” can be critical for discovery. This gives in reality more agency to a human, allowing them to modify their degree of escapism and its fine characteristics when they think this is appropriate. E.g., if you are stuck for a long time around the same problem, it is wise to switch things up. Take less of a biased walk if you don’t know any better.
Extensions for more realism
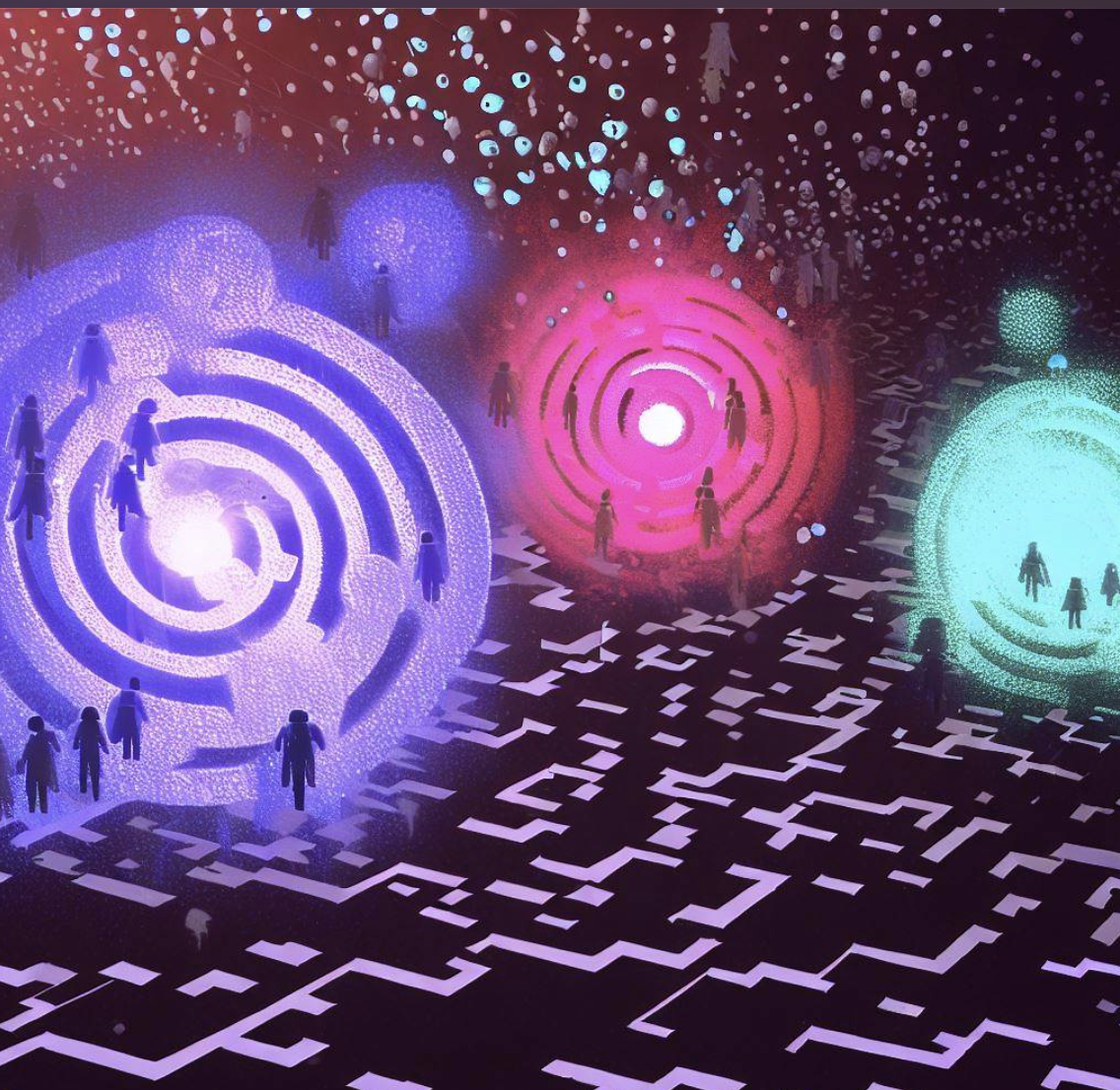
This model does not handle the fact that a discovery by one might bring related discoveries by others - it is only limited to the fact that a discovery by one will often lead to more follow-up discoveries by the same individual. This model can easily be modified to handle this however. Beyond escapism, we may add a parameter affecting the "follower" attribute of a researcher. The followers will try to catch up with the researcher, by performing a biased random walk towards the other researcher, sort of like a one-sided gravitational attractor. This can be implemented beautifully, by adding gravitational forces biasing the walk, with the "discoverers" having larger mass and therefore exerting greater force. The time it takes to catch up is then expected to be proportional to their distance and the obstacles in between.
This model does not model collaboration. This can also be modeled as a group of particles having a stronger gravitational pull on each other than average.
As mentioned in the implications, more realistically, humans perform an evolving random walk, whose biases are changing.
An interesting question is if adding dimensions would increase realism. Note that higher dimensions are characterized by various counter-intuitive phenomena.